How MadKudu makes Salesforce Einstein smarter

...Or why Salesforce Einstein won't be the next IBM Watson. Is the AI hype starting to wither? I believe so, yes. The reality of the operational world is slowly but steadily catching up with the idyllic marketing fantasy. The report Jeffries put together challenging IBM Watson proves alarm bells are ringing. The debacle of the Anderson implementation goes to show how marketing promises can be unrealistic and the downfall will be dreadful. With that said, not all is lost as we keep learning from our past mistakes. Being part of the Salesforce Einstein focused incubator, we are witnessing first-hand how the CRM giant is looking to succeed where Watson and others are struggling. Hopefully, these insights can help others rethink their go-to-market strategy, in an era of unkept commitments.
Salesforce, a quick refresher
A few weeks ago, I was being interviewed for an internal Salesforce video. The question was "how has the Salesforce eco-system helped your startup?". To contextualize my thoughts it's important to know that while Salesforce is one of our main integrations, we consider it as an execution platform among others (Segment, Marketo, Intercom, Eloqua...). I've always admired Salesforce for its "platform" business model. Being part of the Salesforce ecosystem facilitated our GTM. It gave MadKudu access to a large pool of educated prospects. However, I believe the major value add for startups is the focus induced by working with Salesforce customers. Since Salesforce is a great execution platform there are a plethora of applications available addressing specific needs. This means, as a startup, you can focus on a clearly defined and well-delimited value proposition. You can rely on other solutions to solve for peripheral needs. As David Cohen reminded us during our first week at Techstars, "startups don't starve, they drown". Salesforce has helped us stay afloat and navigate its large customer base.
What is Salesforce Einstein?
I'm personally very excited about Salesforce Einstein. For the past 5 years, I've seen Machine Learning be further commoditized by-products such as Microsoft Azure, Prediction.io... We've had many investors ask us what our moat was given this rapid democratization of ML capabilities and our answer has been the same all along. In B2B Sales/Marketing software, pure Machine Learning should not be considered a competitive advantage mainly because there are too few data sets available that require non-generic algorithms. The true moat doesn't reside in the algorithms but rather in all the aspects surrounding them: feature generation, technical operationalization, prediction serving, latency optimization, business operationalization... The last one being the hardest yet the most valuable (hence the one we are tackling at MadKudu...).Salesforce Einstein is the incarnation that innovation will be in those areas since anyone can now run ML models with their CRM.
We've been here before
Just a reminder, this is not a new thing. We've been through this not so long ago. Remember the days when "Big Data" was still making most of the headlines on Techcrunch? Oh, how those were simpler times...
Big Data vs Artificial Intelligence search trends over the past 5 years there were some major misconceptions as to what truly defined Big Data especially within the context of the Enterprise. The media primarily focused on our favorite behemoths: Google, Facebook, Twitter, and their scaling troubles. Big data became synonymous with Petabytes and unfathomably large volumes of data more generally. However, scholars defined a classification that qualified data as "big" for 3 reasons:- volume: massive amounts of data that required distributed systems from storage to processing- velocity: quickly changing data sets such as product browsing. This meant offline/batch processing needed an alternative- variety: data originating from disparate sources meant complex ERDs had to be maintained the Enterprise, volume was rarely the primary struggle. Velocity posed a few issues to large retailers and companies like RichRelevance nailed the execution of their solution. But the main and most challenging data issue faced was with a variety of data.
What will make Salesforce Einstein succeed
Einstein will enable startups to provide value to the Enterprise by focusing on the challenges of:- feeding the right data to the platform- defining a business playbook of ways to generate $$ out of model predictionsWe'll keep the second point for a later blog post but to illustrate the first point with DATA, I put together an experiment. I took one of our customers' dataset of leads and opportunities. The goal was to evaluate different ways of building a lead scoring model. The objective was to identify patterns within the leads that indicated a high likelihood of it converting to an opportunity. This is a B2B SaaS company selling to other B2B companies with a $30k ACV. I ran an out-of-the-box logistic regression on top of the usual suspects: company size, industry, geography, and Alexa rank. For good measure, we had a fancy tech count feature that looked at the number of technologies that could be found on the lead's website. With about 500 opportunities to work on, there was a clear worry about overfitting with more features. This is especially true since we had to dummy the categorical variables. Here's how the regression performed on the training data (70% of the dataset) vs the test dataset (30% that were not used for training and ensuring if a company is part of the training it is not part of testing - see we did not fool around with this test)
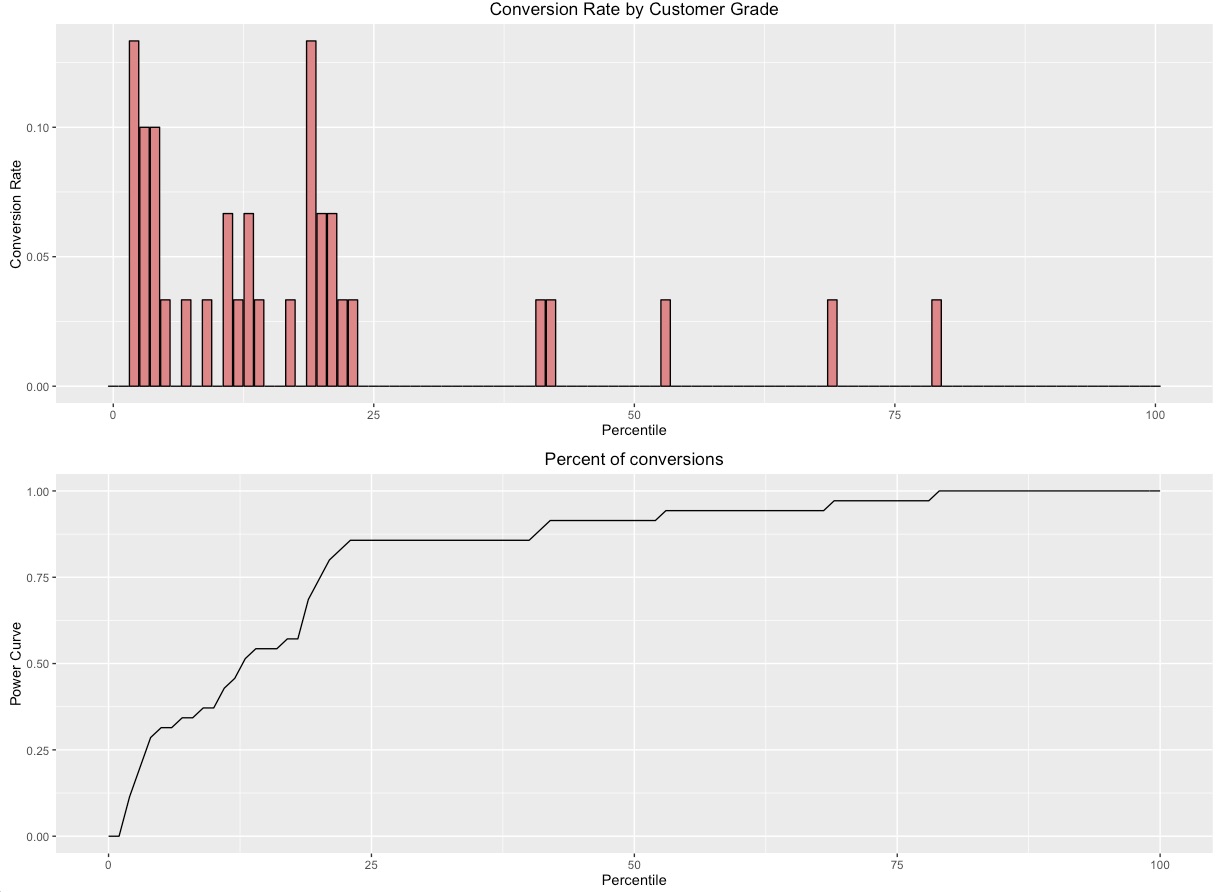
Not bad right?! There is a clear overfitting issue but the performance is not dreadful apart for a blob in the centerNow we ran the same logistic regression against 2 feature: predicted number of tracked users (which we know to be highly tied to the value of the product) and predicted revenue. These features are the result of predictive models that we run against a much larger data set and take into account firmographics (Alexa rank, business model, company size, market segment, industry...) along with technographics (types of technologies used, number of enterprise technologies...) and custom data points. Here's how the regression performed:
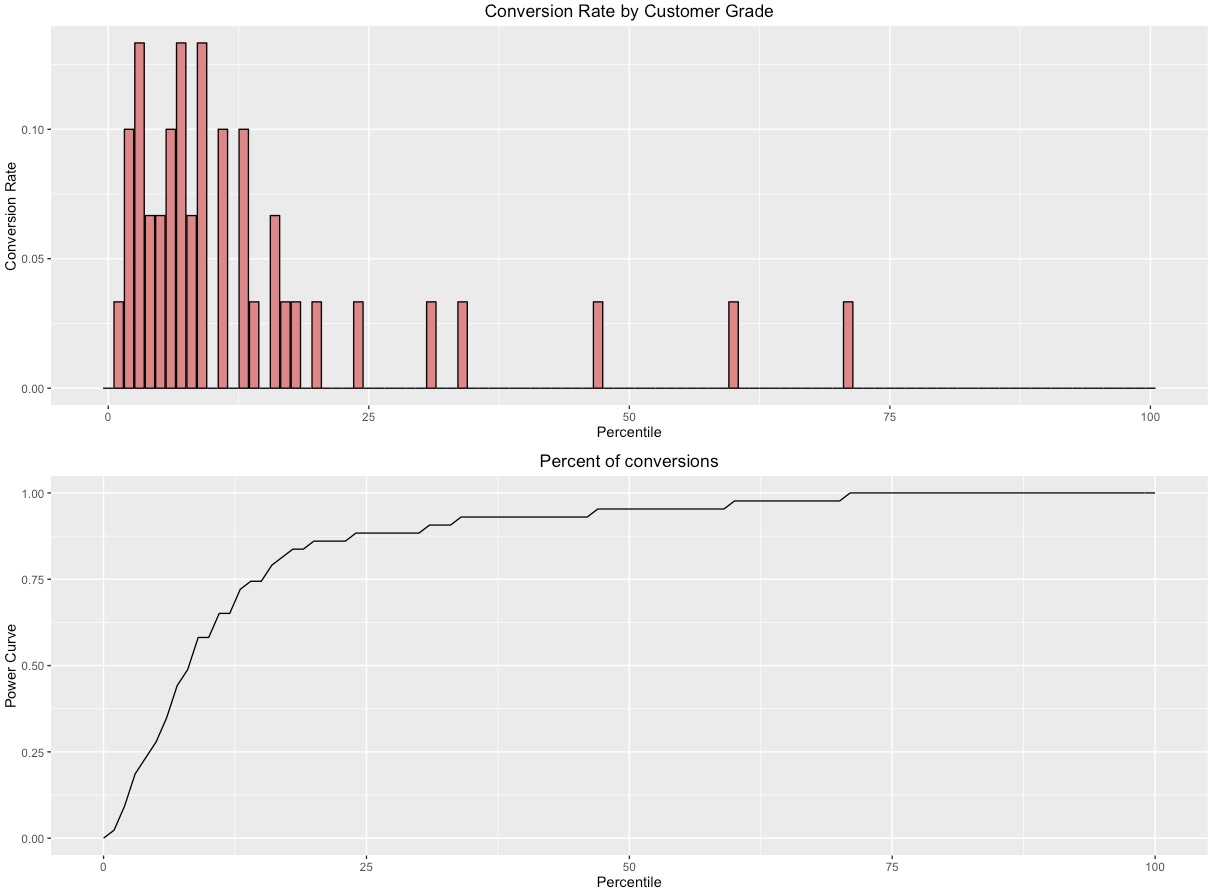
Quite impressive to see how much better the model performs with fewer features. At the same time, we are less running the risk of overfitting as you can see. The TL;DR is that no amount of algorithmic brute force applied to these B2B data sets will ever make up for appropriate data preparation. In essence, Salesforce is outsourcing the data science part of building AI-driven sales models to startups who will specialize in verticals and/or use-cases. MadKudu is a perfect illustration of this trend. The expert knowledge we've accumulated by working with hundreds of B2B SaaS companies is what has enabled us to define these smart features that make lead scoring implementations successful. So there you have it, MadKudu needs Salesforce to focus on its core value and Salesforce needs MadKudu to make its customers and therefore Einstein successful. That's the beauty of a platform business model. I also strongly believe that in the near future there will be a strong need for a "Training dataset" marketplace. As more of the platforms make ML/AI functionalities available, being able to train them out-of-the-box will become an important problem to solve. These "training datasets" will contain a lot of expert knowledge and be the result of heavy data lifting. Feel free to reach out to learn more. To be clear, we are not dissing on IBM's technology which is state of the art. We are arguing that out-of-the-box AI has been overhyped in the Enterprise and that project implementation costs have been underestimated due to a lack of transparency on the complexity of configuring such platforms.