One Lead Score, to Rule Them All?
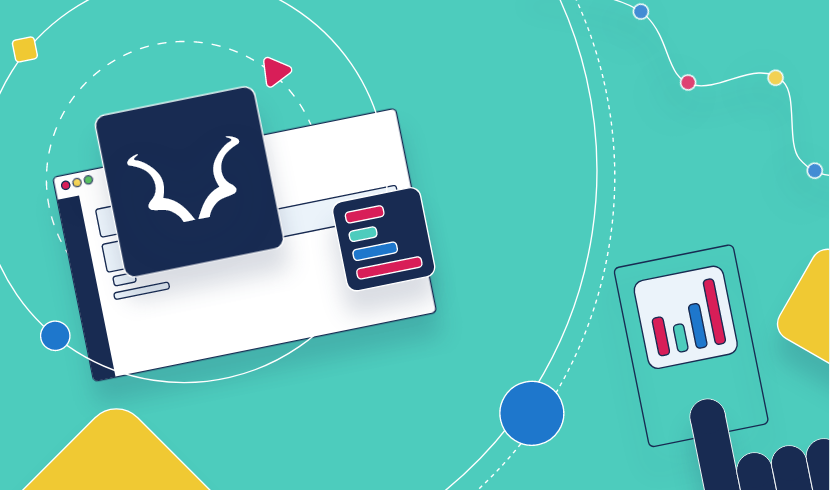
“Having more than one lead score is too complicated for sales.”
“It’s easier to operationalize processes with a single lead grade.”
Sound familiar? We often hear statements like these from marketers. We understand the desire to simplify processes and streamline workflows. Who doesn’t want to make things less complicated? And, much of today’s technology limits what can be achieved with scoring.
We’re not saying that having a single lead score is the wrong answer for everyone. It’s critical to think about your business problem first. Predictive models and multiple scores may not be the solution for you, depending on the challenge at hand. If your goal is simply to prioritize leads based on a single funnel, one lead score may work.
While adding increased complexities isn’t always the answer, having one lead scoring model and grade typically ignores critical nuances important to driving the right business outcomes. Suppose you’re trying to analyze the data and make informed decisions based on different segments, funnels, go-to-market motions, geographies, and more. In that case, you likely need separate demographic and behavioral scores.
Why there isn’t one lead score to rule them all
To make informed sales and marketing decisions, you need an increased level of granularity beyond a single letter grade (A-E). Mashing together demographic, firmographic, technographic, and behavioral attributes doesn’t provide the whole story. Having a singular lead grade acts more like a summary but doesn’t speak to the granularity behind it.
For example, the letter grade of “A” sounds great, right? Let’s take a look deeper.
It can mean that a lead has a very high likelihood to buy (behavioral) and very good fit (demographic) OR that they have a high likelihood to buy and very good customer fit OR that they have a very high likelihood to buy and good customer fit.

For example, a lead with a letter grade of “A” may be extremely active on your site, booking a meeting, reading blog content, and more, but they may not be the best fit (company size and use of older technology) for your company.
.png)
Each of these permutations has a different impact and drives unique sales and marketing actions. The letter grade provides a picture from a distance, but to zoom in and truly see the details, separate fit, and likelihood to buy scores are a must.
As a marketer, what does this mean for you?
Understanding the details behind a letter grade enables you as a marketer to drive the right actions throughout the customer journey.
Acquisition
For acquisition programs, it’s key to focus on driving very good-fit leads. At the top of the funnel, successful marketers optimize their campaigns to find the right profile of people for their business. Evaluating the profiles of leads you are bringing in through acquisition campaigns helps determine what levers need to be pulled and what changes need to be made across platforms and channels to reach your ideal customers.
Post-Acquisition
Moving down the funnel, post-acquisition, focusing on the likelihood to buy (or behavioral) score is essential. At this stage in the buyer’s journey, you can no longer impact customer fit. You’ve got the leads; now you have to figure out what to do with them. Successful marketers build nurture campaigns and programs to progress people through the funnel and encourage them to take the right actions. Understanding a lead’s likelihood to buy and what actions they have taken will help you craft more informed programs that drive conversions and, ultimately, opportunities.
.png)
How does this impact the sales team?
Now that we’ve covered how these nuances affect marketing, let’s look at sales.
Lower-Quality Leads
Having only one score often results in sales receiving low-quality leads who don’t fit the ICP.
For example, sending a sales rep a lead with a letter grade of “B” sounds like it should be a fairly high-quality lead. However, behind that letter grade are many different permutations, one being only a medium fit (demographic score) but a high likelihood to buy (behavioral score).
The high likelihood to buy score could be from taking action like requesting a demo, boosting the overall letter grade. However, a medium fit score means that the lead may not be the right profile to speak with sales (irrelevant industry, tech that doesn't integrate well with your product, etc.). Instead, they might be a better fit for automated nurture or self-serve. Separating the scores provides sales with more clarity on who they should talk to based on both demographic and behavioral factors.
.png)
Additional Context
Separate scores also enable marketing and operations teams to provide further context to fuel sales outreach.
When providing separate scores to sales, it is critical to include signals or context behind the scores. Sales teams don’t always care about the number or letter of the score (or the number of flames), but having visibility into why someone is scored a certain way and what they have done is essential in crafting personalized outreach.
For example, let’s say you work at a B2B tech company selling into the marketing space. A lead with a very high fit score may have some of the following signals:
.png)
This information provides sales with key insights into why the score is high and why the lead is relevant to them (relevant role, use of complementary technology, etc.).
Providing behavioral context empowers reps to reach out with the right message. Looking at the signals below, a sales rep could follow up with content related to the webinar, ask if they have any questions about the pages they've viewed, or share relatable stories of customers with similar challenges.
.png)
To make scores relevant, there needs to be additional context and information provided. Building trust and transparency with sales teams requires more than saying this lead is an “A” or “B” so you should follow up.
Lead Scoring For Complex B2B Organizations
One lead score also doesn’t speak to the broader complexities of a B2B organization. What may be predictive of a very good lead or account for an enterprise motion doesn’t translate to a product-led motion. The same rings true when considering nuances across geographies.
Predictive scoring models are optimized for specific outcomes. For example, a model may be optimized for opportunities with a probability of 60% or higher and an amount of $100,000 or higher. An individual from a large public company with a large budget would have a very high fit score.
An individual at a small company with a small budget will have a low fit score. However, they may actually be ready to use the product and swipe their credit card, making them a great fit for your company’s PLG motion. A separate model (and score) is necessary to evaluate the fit and likelihood to buy within a velocity motion.