How Lucid Increased Pipeline +60% by Optimizing Multiple Funnels
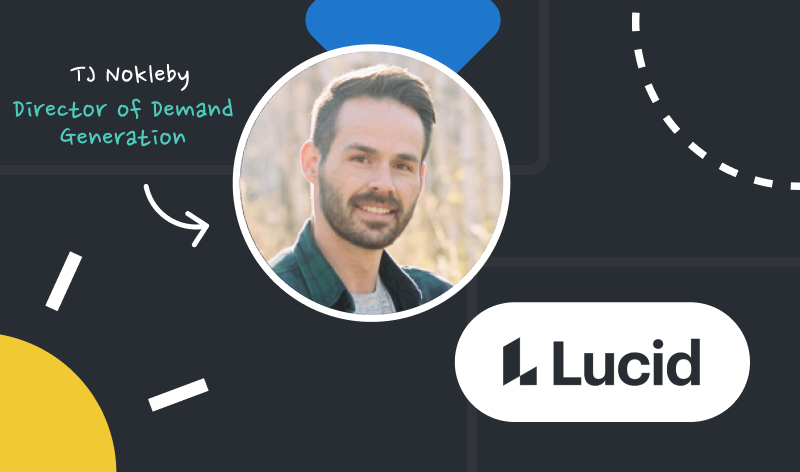
+80%
Improved PQL attempt rates
+60%
Increase in pipeline from PQLs
+20%
Increase in ACV
MadKudu helped Lucid optimize 4 GTM motions
In today’s market, revenue leaders are looking for new ways to reliably generate pipeline.
We sat down with TJ Nokleby, Director of Demand Generation at Lucid to hear how they used MadKudu to first optimize their PLG motion, and then to run successful account-based, expansion, and inbound marketing plays.
The initial challenge
When Lucid first came to MadKudu, they had a simple goal: generate more pipeline from their 1 million new product sign-ups each month.
However, one million users paired with an existing base of tens of millions of users, is an overwhelming amount of data to sift through.
It was impossible to know which of the millions of users in the database were most likely to turn into paying customers. They needed a quick and accurate way to pinpoint who their revenue team should target.
Struggling with homegrown solution
Before MadKudu, Lucid failed to create a scoring system that increased the revenue generated from users.
Unreliable infrastructure
Lucid was using Marketo, but marketing automation software is not built for updating millions of records they were getting from both inbound leads and product usage. When they tried to process large requests, the system would struggle.
Time consuming to make changes
There were very capable analysts and engineers on the team, but there was no way to iterate quickly on the model without introducing risk. It took 100+ hours for an analyst to review and update the model, not including meeting with other stakeholders to discuss and collaborate on the changes.
Lack of visibility for sales
Sales didn’t trust product qualified leads (PQLs) and thought they were a black box because they were not given any context as to what made them qualified. This led to them only reaching out to 30% of PQLs.
Product Qualified Leads in MadKudu
Lucid was able to deploy a better solution in partnership with MadKudu.
They integrated their product data with MadKudu’s platform to see which user activities correlated to revenue, and the platform built a scoring model based on this information.
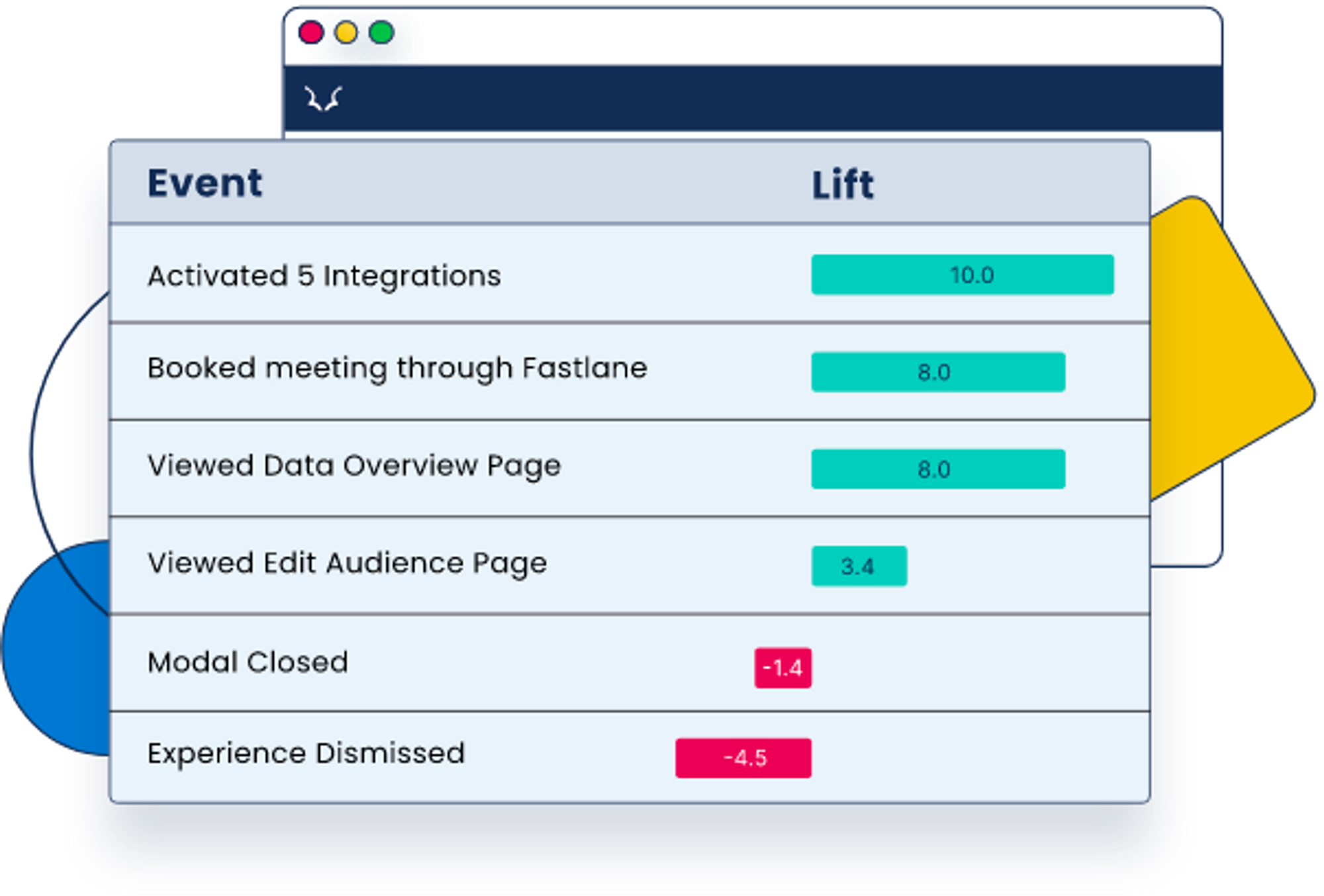
The scores were populated in Salesforce, along with the key buying signals, which allowed sales to send personalized outreach to the best fit leads.
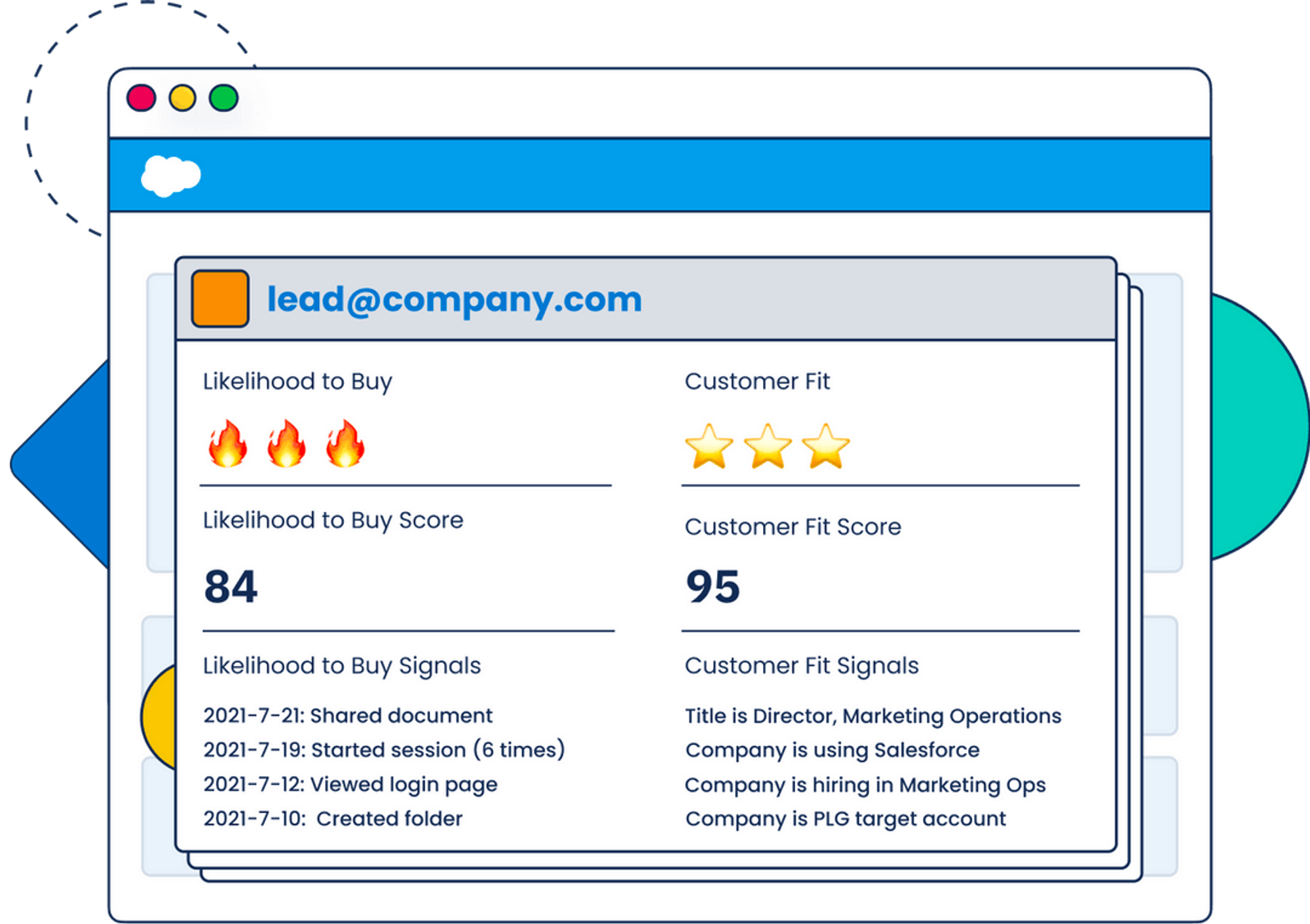
The marketing team then got feedback from sales to see if there were any signals they were missing, or ones that were over-indexed. That way the model could be optimized in real time based on feedback.
The Results
The ability to rapidly iterate on signals and methodology was a huge bonus, iteration time for a model went from 1 quarter to 1 week, saving the team hundreds of hours.
The reps felt heard and were able to see their feedback come to life which led to an 80% increase in PQL attempt rates.
Seeing what activities a user had completed recently in addition to key traits about them made them more effective at engaging, and marketing sourced pipeline from PQLs increased by 60%.
“We use MadKudu to identify insights in our data and focus on what matters most, which is absolutely critical to the success of our business.”
The screenshot below is a snapshot from Lucid’s CRM. At the lead level, you can see the user score, as well as behavior signals that give context as to why this lead is product qualified.
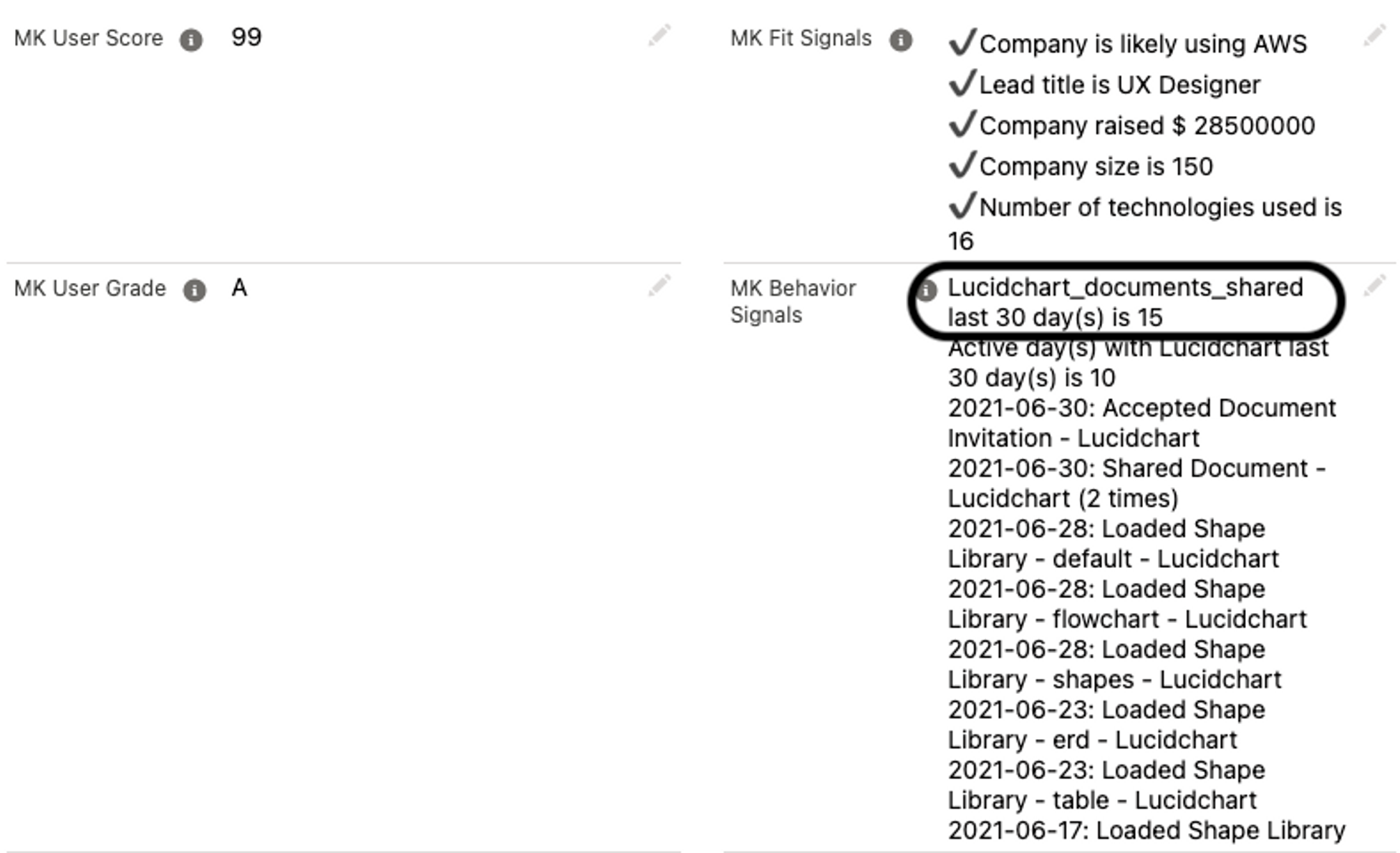
ABM Strategy
Now that Lucid’s PQL system had drastically improved, they could turn their attention to other revenue streams.
Their competitors were starting to sell top-down, and it was time to focus less on transaction selling and start landing bigger deals.
They needed a way to prioritize accounts to know which ones are ready to buy, as well as which ones they needed to put more marketing dollars against.
The challenge
Each seller owned a lot of accounts, around 100-1000+ at any given time. Researching these accounts took a lot of time, and was fragmented across different tools.
Account selection was subjective, and chosen with limited data in partnership between marketing and sales.
Data backed solution
Using MadKudu, Lucid was able to aggregate user-level insights and layer in account-level insights through enrichment to start collecting feedback from a pilot group of sellers.
Since sellers needed additional context to have strategic conversations, the team at Lucid found ways to surface clues from MadKudu’s data points about what might be happening at the account.
For example, if a company is hiring in engineering, they’re using GCP, and they’re using cloud shapes, there’s likely a use case for their Lucidscale product.
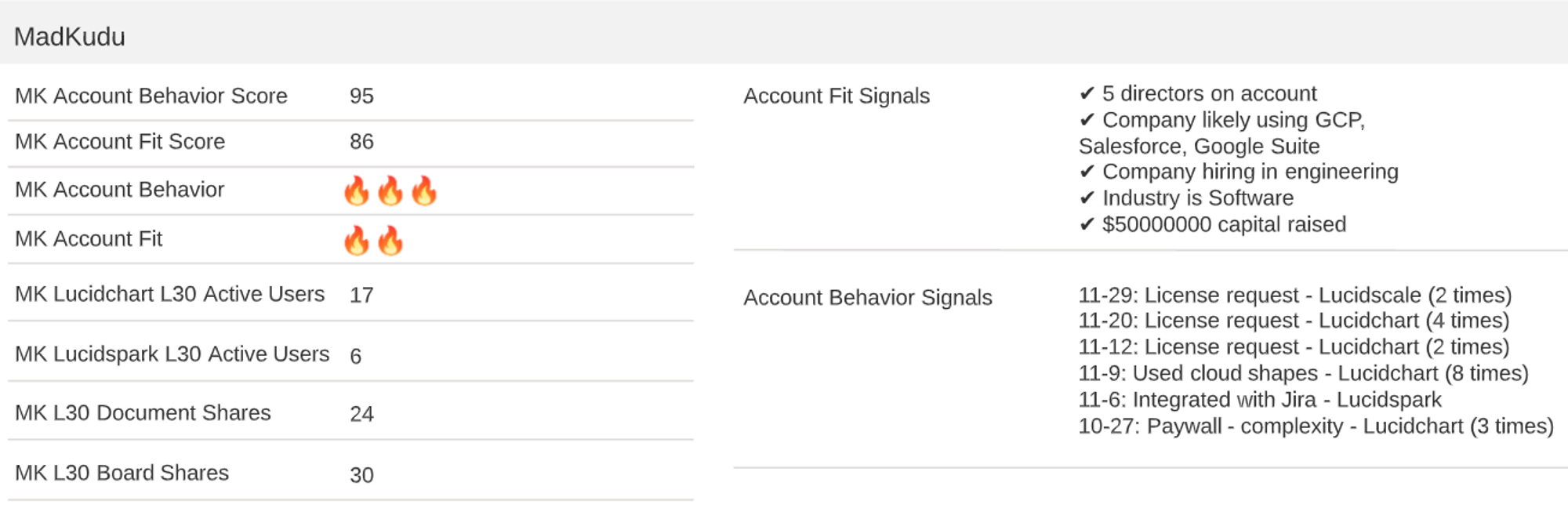
Armed with that knowledge, a seller who owns this account can find the right users on the account, and start a conversation, with context.
This was a massive way for marketing to build trust with sales, and it was all automated and right in Salesforce.
By identifying the right accounts to reach out to, Lucid was able to increase average customer value (ACV) by +20%.
Expanding this strategy to existing customers
Lucid has three products, each with a few subscription levels, so there is a lot of opportunity for expansion and cross-sell plays.
Using MadKudu, Lucid was able to combine product behavior, such as a license request for a new product, and profile data, such as the account using a direct competitor, to figure out which play they can run.
This allowed the team to contact the account with messaging that’s highly relevant to the actions they are taking.
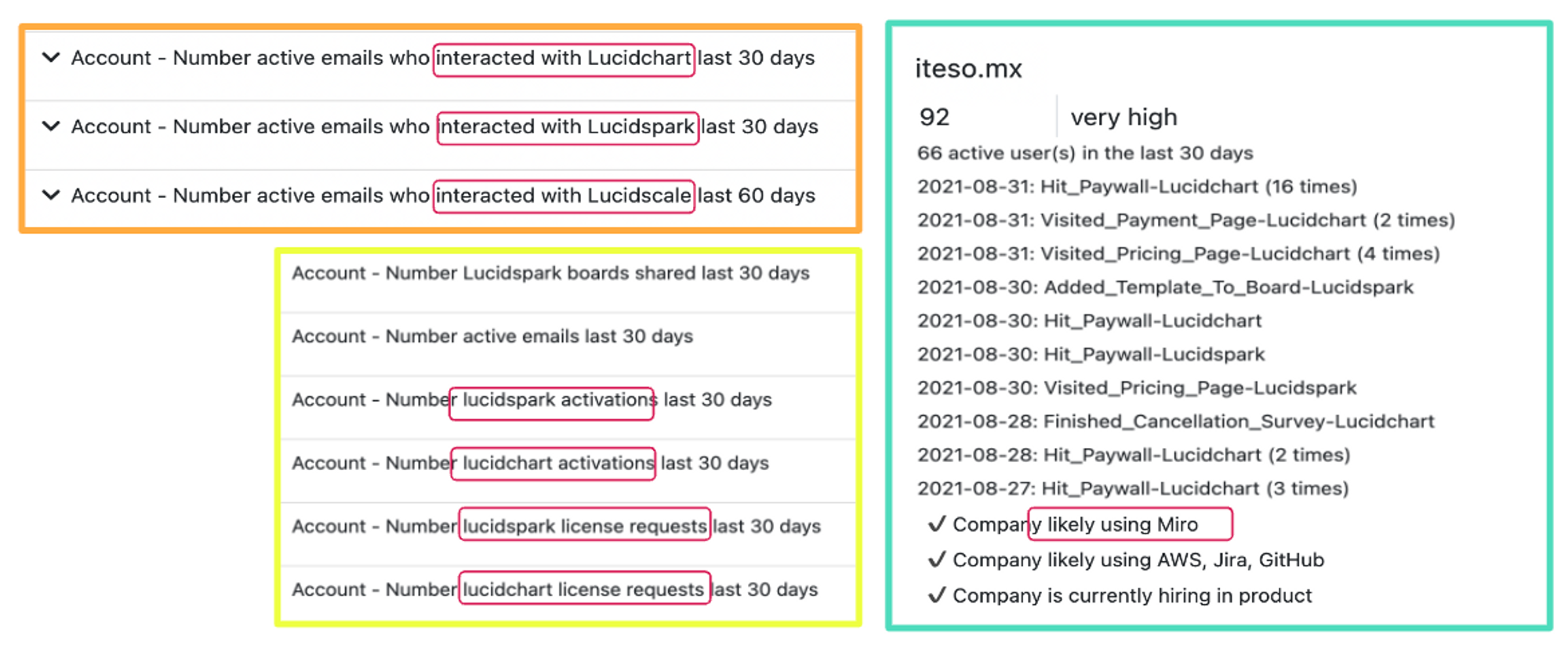
By having customized plays, Lucid was able to increase touches to high-scoring accounts by 25%, and these accounts made up 80% of total revenue.
The feedback from sales was resoundingly positive. They were being served valuable accounts they would have likely overlooked without MadKudu.

The Newest Goal: Optimizing Inbound
Once Lucid had seen success scoring leads and accounts for the sales team to reach out to, they turned their attention to use this same data on the marketing side.
Since they already knew which target accounts they wanted to nurture further, they were able to build higher intent audiences to orchestrate intentional plays.
They used MadKudu data to determine when they should enroll people from an account into a nurture, an ad audience, or when to send them a touch via Sendoso.
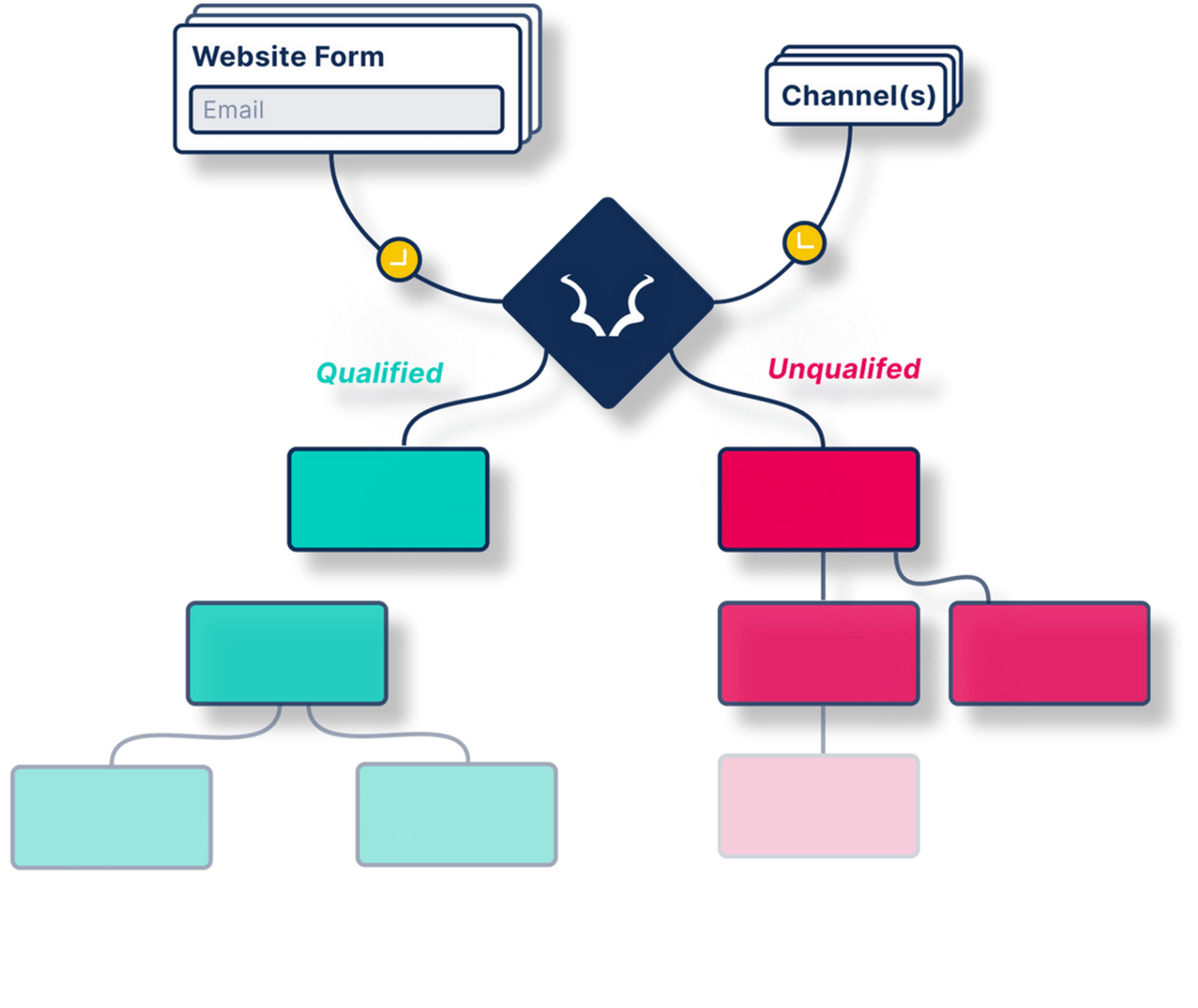
By using the same data as the sellers, they were able to really operate as one team. By automating a lot of the analysis needed to run campaigns, they were able to better support sellers in generating pipeline.